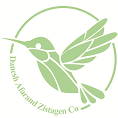
@2024 Afarand., IRAN
ISSN: 2538-4384 Geographical Researches 2021;36(2):191-203
ISSN: 2538-4384 Geographical Researches 2021;36(2):191-203
Detection of Surface Temperature Changes Using Satellite Images and Real Data and its Relationship with the Covered Vegetation in the Southern Part of the Lake Urmia
ARTICLE INFO
Article Type
Original ResearchAuthors
Mikaili HajiKandi Kh. (1)Sobhani B. (*1)
Vramesh S. (2)
(1) Department of Geography, Faculty of Social Sciences, Mohaghegh Ardabili University, Ardabil, Iran
(2) Department of Science and Forest Engineering, Faculty of Agriculture and Natural Resource, University of Mohaghegh Ardabili, Ardabil, Iran
Correspondence
Address: Department of Geography, Faculty of social Sciences, Mohaghegh Ardabili University, Daneshgah Street, Ardabil, Iran. Postal Code: 5619911367Phone: +98 (45) 33596107
Fax: +98 (45) 31505981
sobhani@uma.ac.ir
Article History
Received: November 9, 2020Accepted: January 25, 2021
ePublished: June 16, 2021
BRIEF TEXT
Detection of the weather changes in the past is of great importance in the future weather forecast. Remote sensing provides the opportunity to estimate land surface temperature in each region accurately.
Environmental impacts are the most influential factors in developing countries because agriculture-oriented economies are getting more prevalent. Akbari et al. (2016) studied the relationship between land surface temperature and land-use changes in the Taleghan watershed in 1987 and 2010 using Landsat images. They concluded that residential areas and bare lands have the highest temperature in comparison to other land uses. Chaudhuri & Mishra (2016) analyzed the changes in the land surface temperature according to the changes in land use and vegetation. Their result shows that changes in the land use increases the temperature. Kazemi Garajeh et al. (2020) used MODIS satellite images in various types of the split-window algorithms in order to estimate land surface temperature. According to their results, the lowest temperature degrees are recorded in the mountains with vegetation cover while the highest ones are recorded in the plains without dense vegetation cover.
This study aimed to evaluate the land surface temperature in the southern part of Urmia Lake using Landsat data.
The current research is empirical that is descriptive-analytical in terms of its methodology.
The current research is carried out in southern parts of Urmia Lake from 2000 to 2017
The sampling method and the number of samples are not mentioned.
The indices of NDVI and NIR extracted from Landsat images were calculated in ArcGIS10 and ENVI5.1 software.
Each class of land cover area in the studied area is needed to estimate the region land cover changes. The results show a huge difference in the classes' area between 2017 and 2000. The rising and falling changes are significant in some cases. The detailed information of each year in the studied area is presented in Table 1.Each class of land cover area in the studied area is needed to estimate the region land cover changes. The results show a huge difference in the classes' area between 2017 and 2000. The rising and falling changes are significant in some cases. The detailed information of each year in the studied area is presented in Table 1. According to the results of Table 1, the overall accuracy and Kappa coefficient are more than 90% and 80%, respectively. Accordingly, this method is proper in the production of the information and it was accurate enough in terms of showing the land cover changes in the studied area. The results of land surface temperature extraction in the studied area in 2000 and 2017 are presented in Figure 3. The land surface temperature in 2000 varies from 18 to 48 ˚C, while it varies from 25 to 57˚C in 2017. Those lands covered by water, irrigated farms, and gardens had the lowest temperature in both years, while rain-fed lands, rangelands, and bare lands and residential areas had the highest temperature in both 2000 and 2017. The relationship between land surface temperature and NDVI, on the other hand, was studied. Figure 4 shows that the vegetation cover in the studied area belongs to the irrigated agricultural lands and gardens. Land surface temperature in such areas is less than areas without vegetation because of the light reflection of the leaves. Lands without vegetation cover absorb light and have higher temperatures during the day. In order to show the relationship between land surface temperature and vegetation, some spots were selected randomly and were studied. Then, the regression equation showed that there is a negative relationship between these two variables (Figure 5). Validation of the results of land surface temperature, their changes and the real temperature was done using the Mann-Kendall test in each station. Graph 1(a) shows the changes in the maximum temperatures in Maraghe station from 2007 to 2019 that had increased significantly. Although there were some fluctuations in this trend before 2007, it was all increasing from 2007 to 2019. The changes in the minimum temperature in the mentioned station is presented in Graph 1 (b). The trend was rising from 1995 to 1997 when the maximum temperature trend fell down. The minimum temperature trend increased significantly from 2016 to 2019.Graph 9 shows the relationship between the measured data and the results of LST in 2017 using linear regression. R2 coefficient is equal to 72% that shows a strong relationship between these two factors. As some of the stations were founded in 2000, the relationship between data was not possible in 2000. To sum up, encountering the shortage of meteorological data, satellite images are more efficient.
The results of the current study are consistent with the results of Jouybari Moghadam et al. (2015) and Feizizadeh et al. (2016) about the impact of land surface type on the temperature and its correlation with NDVI. Yue et al. (2007) showed that LST and NDVI are correlated and land surface temperature is influenced by the land cover especially the vegetation. Better function of the split-window algorithm in the calculation of the land surface temperature approves the results of the current study [Solanky et al., 2018].
Using the satellite images, it is suggested to use the methods approved by other researchers because more accurate data will be provided. The more accurate extracted data from satellite images can be generalized in other similar regions.
Lack of enough satellite images is one of the limitations that makes it difficult to select a collection of images at proper times and locations. In addition, the lack of enough measured data in some regions is very problematic.
Use of the satellite images to evaluate climate factors is of great importance particularly in regions without enough data. Land surface temperature is one of the most important factors in relation to agricultural studies and environmental planning. The evaluation of changes in the land cover and vegetation highlights the importance of land surface temperature. Using this factor, long-term climate changes can be detected.
We thank the meteorology organization for the provision of the needed data.
None.
None.
None.
TABLES and CHARTS
Show attach fileCITIATION LINKS
[1]Afzan Buyadi S N, Naim W-M, Misni A (2013). Impact of land use changes on the surface temperature distribution of area surrounding the national botanic garden. Shah Alam. Procedia-Social and Behavioral Sciences. 101:516-525.
[2]Akbari E, Ebrahimi M, Fiezizadeh B, Nezhadsoleimani H (2016). Evaluating land surface temperature related to the land use change detection by satellite image (Case study: Taleghan Basin). Geography and Environmental Planning Journal. 4(60):151-170. [Persian]
[3]Ansari M, Noori Gh, Fotoohi S (2016). Investigation of temperature precipitation and flow trend using nonparametric mankendall (Case study: Kaju River in Sistan and Baluchestan). Journal of Watershed Management Research. 7(14): 152-158. [Persian]
[4]Arvin A (2019). Land surface temperature detection using of satellite images. Journal of Natural Environmental Hazards. 8(19):91-102. [Persian]
[5]Aslami F, Ghorbani A, Sobhani B, Panahandeh M (2015). Comparing artificial neural network, support vector machine and object-based methods in preparation land use/cover mapsusing landSat-8 images. RS and GIS for Natural Resources. 6(3):1-14. [Persian]
[6]Asgarzadeh P, Darvishi Boloorani A, Bahrami H.A, Hamzeh S (2016). Comparison between land surface temperature estimation in single and multi-channel method using landsat images 8. Journal of Rs and Gis for Natural Resources. 7(3):18-29. [Persian]
[7]Ayanlade A, Howarda M T (2019). Land surface temperature and heat fluxes over three cities in Niger Delta, Journal of African Earth Sciences. 151:54-66.
[8]Chaudhuri G, Mishra N B (2016). Spatio-temporal dynamics of land cover and land surface temperature in Ganges-Brahmaputra delta: A comparative analysis between India and Bangladesh, Applied Geography. 68:68-83.
[9]Chen X L, Zhao H M, Li P X, Yin Z Y (2006). Remote sensing image-based analysis of the relationship between urban heat island and land use/cover changes. Remote Sensing of Environment. 104(2):133-146.
[10]Choudhury D, Das K, Das A (2019). Assessment of land use land cover changes and its impact on variations of land surface temperature in Asansol-Durgapur Development Region. The Egyptian Journal of Remote Sensing and Space Sciences. 22(2):203-218.
[11]Feizizadeh B, Didehban Kh, Gholamnia Kh (2016). Extraction of land surface temperature (LST) based on lndsat satellite images and split window algorithm. Study area: Mahabad Catchment. Journal of Geographical Data (SEPEHR). 25(98):171-181. [Persian]
[12]Ghorbannia Kheybari V, Mirsanjari M, Liaghati H, Armin M (2017). Estimating land surface temperature of land use and land cover in dena county using single window algorithm and landsat 8 satellite data. Environmental Sciences Journal. 15(2):55-74. [Persian]
[13]Hejazizadeh Z, Ziaeian P, Shirkhani A (2013). Estimation of Surface temperature using thermal-band data in west of Tehran province and Qazvin. Geography. 11(38):33-49. [Persian]
[14]Hengl T, Heuvelink G B M, Tadic M P (2012). Spatio-temporal prediction of daily temperatures using time-series of MODIS LST images. Theoretical and Applied Climatology. 107:265-277.
[15]Jahandideh M, Shirvani A (2012). Trend analysis for precipitation time sub-series in Fars province. Water Engineering Journal. 5(12):73-84. [Persian]
[16]Jouybari Moghaddam Y, Akhoondzadeh M, Saradjian M R (2015). A novel method for retrieving land surface emissivity from landsat-8 satellite data based on vegetation index. Jurnal of geomatics science and technology. 5(1):175-187. [Persian]
[17]Kazemi Garajeh M, Salmani B, Feizizadeh B (2020). Evaluating the types of split window algorithms for calculating the land surface temperature to determine the best algorithm for MODIS sensor images. RS and GIS for Natural Resources. 11(2-39):106-127. [Persian]
[18]khoshhal dastjerdi J, ghavidel Rahimi Y (2008). Application of Mann-Kendall nonparametric test in estimating temperature changes, Geographic Space. 8(22): 21-38. [Persian]
[19]Klok L, Zwart S J, Verhagen H, Mauri E (2012). The surface heat island of Rotterdam and its relationship with urban surface characteristics. Resources, Conservation and Recycling Journal. 64:23-29.
[20]Meng X, Li H, Du Y, Liu Q, Zhu J, Sun L (2016). Retrieving land surface temperature from landsat 8 TIRS data using RTTOV and ASTER GED. IEEE International Geoscience and Remote Sensing Symposium (IGARSS). 4302-4305.
[21]Morawitz D F, Blewett T M, Cohen A, Alberti M (2006). Using NDVI to assess vegetative land cover change in central Puget sound. Environmental Monitoring and Assessment Journal. 114(1-3):85-106.
[22]Nichol J E, Hang T P (2012). Temporal characteristics of thermal satellite images for urban heat stress and heat land mapping, ISPRS Journal of Photogrammetry and Remote Sensing. 74:153-162.
[23]Rogan J, Ziemer M, Martin D, Ratick S, Cuba N, De Lauer V (2013). The impact of tree cover loss on land surface temperature: A case study of central Massachusetts using landsat thematic mapper thermal data. Applied Geography Journal. 45:49-57.
[24]Solanky V, singh S, katiyar S (2018). Land Surface Temperature Estimation Using Remote Sensing Data. Hidrologic Modeling Journal. 343-351.
[25]Syvitski J P (2008). Deltas at risk. Sustainability Science Journal. 3(1):23-32.
[26]Weng Q, Lu D (2008). A sub-pixel analysis of urbanization effect on land surface temperature and its interplay with impervious surface and vegetation coverage in Indianapolis, United States. International Journal of Applied Earth Observation and Geoinformation. 10(1):68-83.
[27]Xiong Y, Huang Sh, Chen F, Ye H, Wang C, Zhu C (2012). The impacts of rapid urbanization on the thermal environment: A remote sensing study of Guangzhou. South China. Remote Sensing Journal. 4(7):2033-2056.
[28]Yue W, Xu J, Tan W (2007). The relationship between land surface temperature and NDVI with remote sensing: application to Shanghai Landsat 7 ETM + data. International Journal of Remote Sensing. 28(15): 3205-3226.
[2]Akbari E, Ebrahimi M, Fiezizadeh B, Nezhadsoleimani H (2016). Evaluating land surface temperature related to the land use change detection by satellite image (Case study: Taleghan Basin). Geography and Environmental Planning Journal. 4(60):151-170. [Persian]
[3]Ansari M, Noori Gh, Fotoohi S (2016). Investigation of temperature precipitation and flow trend using nonparametric mankendall (Case study: Kaju River in Sistan and Baluchestan). Journal of Watershed Management Research. 7(14): 152-158. [Persian]
[4]Arvin A (2019). Land surface temperature detection using of satellite images. Journal of Natural Environmental Hazards. 8(19):91-102. [Persian]
[5]Aslami F, Ghorbani A, Sobhani B, Panahandeh M (2015). Comparing artificial neural network, support vector machine and object-based methods in preparation land use/cover mapsusing landSat-8 images. RS and GIS for Natural Resources. 6(3):1-14. [Persian]
[6]Asgarzadeh P, Darvishi Boloorani A, Bahrami H.A, Hamzeh S (2016). Comparison between land surface temperature estimation in single and multi-channel method using landsat images 8. Journal of Rs and Gis for Natural Resources. 7(3):18-29. [Persian]
[7]Ayanlade A, Howarda M T (2019). Land surface temperature and heat fluxes over three cities in Niger Delta, Journal of African Earth Sciences. 151:54-66.
[8]Chaudhuri G, Mishra N B (2016). Spatio-temporal dynamics of land cover and land surface temperature in Ganges-Brahmaputra delta: A comparative analysis between India and Bangladesh, Applied Geography. 68:68-83.
[9]Chen X L, Zhao H M, Li P X, Yin Z Y (2006). Remote sensing image-based analysis of the relationship between urban heat island and land use/cover changes. Remote Sensing of Environment. 104(2):133-146.
[10]Choudhury D, Das K, Das A (2019). Assessment of land use land cover changes and its impact on variations of land surface temperature in Asansol-Durgapur Development Region. The Egyptian Journal of Remote Sensing and Space Sciences. 22(2):203-218.
[11]Feizizadeh B, Didehban Kh, Gholamnia Kh (2016). Extraction of land surface temperature (LST) based on lndsat satellite images and split window algorithm. Study area: Mahabad Catchment. Journal of Geographical Data (SEPEHR). 25(98):171-181. [Persian]
[12]Ghorbannia Kheybari V, Mirsanjari M, Liaghati H, Armin M (2017). Estimating land surface temperature of land use and land cover in dena county using single window algorithm and landsat 8 satellite data. Environmental Sciences Journal. 15(2):55-74. [Persian]
[13]Hejazizadeh Z, Ziaeian P, Shirkhani A (2013). Estimation of Surface temperature using thermal-band data in west of Tehran province and Qazvin. Geography. 11(38):33-49. [Persian]
[14]Hengl T, Heuvelink G B M, Tadic M P (2012). Spatio-temporal prediction of daily temperatures using time-series of MODIS LST images. Theoretical and Applied Climatology. 107:265-277.
[15]Jahandideh M, Shirvani A (2012). Trend analysis for precipitation time sub-series in Fars province. Water Engineering Journal. 5(12):73-84. [Persian]
[16]Jouybari Moghaddam Y, Akhoondzadeh M, Saradjian M R (2015). A novel method for retrieving land surface emissivity from landsat-8 satellite data based on vegetation index. Jurnal of geomatics science and technology. 5(1):175-187. [Persian]
[17]Kazemi Garajeh M, Salmani B, Feizizadeh B (2020). Evaluating the types of split window algorithms for calculating the land surface temperature to determine the best algorithm for MODIS sensor images. RS and GIS for Natural Resources. 11(2-39):106-127. [Persian]
[18]khoshhal dastjerdi J, ghavidel Rahimi Y (2008). Application of Mann-Kendall nonparametric test in estimating temperature changes, Geographic Space. 8(22): 21-38. [Persian]
[19]Klok L, Zwart S J, Verhagen H, Mauri E (2012). The surface heat island of Rotterdam and its relationship with urban surface characteristics. Resources, Conservation and Recycling Journal. 64:23-29.
[20]Meng X, Li H, Du Y, Liu Q, Zhu J, Sun L (2016). Retrieving land surface temperature from landsat 8 TIRS data using RTTOV and ASTER GED. IEEE International Geoscience and Remote Sensing Symposium (IGARSS). 4302-4305.
[21]Morawitz D F, Blewett T M, Cohen A, Alberti M (2006). Using NDVI to assess vegetative land cover change in central Puget sound. Environmental Monitoring and Assessment Journal. 114(1-3):85-106.
[22]Nichol J E, Hang T P (2012). Temporal characteristics of thermal satellite images for urban heat stress and heat land mapping, ISPRS Journal of Photogrammetry and Remote Sensing. 74:153-162.
[23]Rogan J, Ziemer M, Martin D, Ratick S, Cuba N, De Lauer V (2013). The impact of tree cover loss on land surface temperature: A case study of central Massachusetts using landsat thematic mapper thermal data. Applied Geography Journal. 45:49-57.
[24]Solanky V, singh S, katiyar S (2018). Land Surface Temperature Estimation Using Remote Sensing Data. Hidrologic Modeling Journal. 343-351.
[25]Syvitski J P (2008). Deltas at risk. Sustainability Science Journal. 3(1):23-32.
[26]Weng Q, Lu D (2008). A sub-pixel analysis of urbanization effect on land surface temperature and its interplay with impervious surface and vegetation coverage in Indianapolis, United States. International Journal of Applied Earth Observation and Geoinformation. 10(1):68-83.
[27]Xiong Y, Huang Sh, Chen F, Ye H, Wang C, Zhu C (2012). The impacts of rapid urbanization on the thermal environment: A remote sensing study of Guangzhou. South China. Remote Sensing Journal. 4(7):2033-2056.
[28]Yue W, Xu J, Tan W (2007). The relationship between land surface temperature and NDVI with remote sensing: application to Shanghai Landsat 7 ETM + data. International Journal of Remote Sensing. 28(15): 3205-3226.