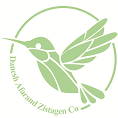
@2025 Afarand., IRAN
ISSN: 2251-8215 Sarem Journal of Reproductive Medicine 2017;1(3):97-104
ISSN: 2251-8215 Sarem Journal of Reproductive Medicine 2017;1(3):97-104
MCV Measurement of Abnormal Red Blood Cells Using Adaptive Neuro-Fuzzy System with Image Processing
ARTICLE INFO
Article Type
Original ResearchAuthors
Babazadeh Khameneh N. (1)Salehian P. (*)
Arabalibeik H. (2)
Setayeshi S. (3)
(*) Sarem Fertility & Infertility Research Center (SAFIR), Sarem Women’s Hospital, Tehran, Iran
(1) Islamic Azad University, Pardis Branch, Tehran, Iran
(2) Science and Technology in Medicine Research Center (RCSTIM), Tehran University of Medical Sciences, Tehran, Iran
(3) Energy Engineering & Physics Department, Amirkabir University of Technology, Tehran, Iran
Correspondence
Article History
Received: February 29, 2016Accepted: June 25, 2016
ePublished: August 15, 2017
BRIEF TEXT
Anemia affects the shape, size, volume, color and number of red blood cells in a given volume. ... [1].
... [2]. The usual methods of diagnosis of anemia, are clinical examinations of the patient and observation of laboratory reports that contains important information including red blood cell count and volume, hemoglobin concentration, etc. All of these calculations are performed by a cell counting system in pathologic laboratories. Thousands of blood samples are taken daily in pathologic laboratories around the world by microscopes and automatic counters. This is usually costly and time consuming. Therefore, automatic detection of abnormal samples will be helpful in reducing time and cost. One of the automated methods is laser scattering, which is used to determine the size of the red blood cell, but has a high cost.
The aim of this study was to measure the average volume of abnormal red blood cells using an adaptive neuro-fuzzy inference system (ANFIS) by image processing.
The initial data used in the study was of a visual type, in the size of 640 × 480 and in the format of the bitmap. The number of slides of normal people was 40 and the number of slides of abnormal individuals was 20. A total of 30-50 images were taken from each slide, and a total of at least 1500 normal images and 1000 unusual images were taken. Nikon's Microscope (Nicon Model NY100) and a Panasonic camera were used to produce images in the laboratory. The camera was connected to the computer from one side to the microscope and on the other side. The lens of the microscope was adjusted on 40 and its magnification was adjusted to 100, and all images were prepared in the same environmental and laboratory conditions. ... [3]. In the beginning, the color images turned to images with gray levels. The gray levels of the images were in the range of 0.225. ... [4]. Threshold was used to convert images with gray levels to a binary image. ... [5].The red cells that stick to the edge of the image are considered unwanted objects and should be removed from the calculations. Therefore, after the gray image became a binary image, these unwanted objects were removed using the image retrieval method. This method uses a hybrid algorithm to quickly recover images [6]. The objects tagging method was used to identify and specify the number of objects in the image. ... [7]. To extract two characteristics of the diameter of the inner cavity of the red blood cell and the outer diameter of the circle, the features of the smallest rectangle of the environment on the RBC were used. Therefore, the average total length and width of this rectangle was considered as the outer diameter of the red blood cell. The closest geometric shape to the human red blood cells is Torus, that this geometric and mathematical simulation can be used to calculate the characteristics of the red blood cell, including volume (Fig. 1) [8]. The Torus can be defined in forms of parameters in which the parameters u and v are in the range ( 0.2 π), R is the distance from the center of the Turus to the center of the tube and r is the radius of the tube: 1) y(u, υ)=(R + r cos υ ) sinus 2) z(u, υ)=r sin υ This can be defined in Cartesian system: 3) (R-√X2+Y2)2+Z2=r2 That it is easier to write: 4) (x2+y2+z2+R2-r2)2=4R2(x2+y2) A is the Torus area which is obtained from the following equation: 5) A=4 π2 Rr=(2πr) (2πR) And V, the volume enclosed by a torus is obtained from the following equation [8]: 6) V=2π2 Rr2=(πr2) (2πR) The volumes outside of the following range are considered unwanted and should be removed from the list before the calculation is made. 7) (a× median)≤Volume≤(b × median) a and b are integers and a <1 and b>1. ANFIS was used to classify blood samples to normal and non-normal groups. The ANFIS system is an adaptive and educable network that is similar in function to the fuzzy inference system. For simplicity we assume that our fuzzy system has two inputs x and y and its output is z. Now if the rules are as follows: 8) Rule1: if x is A1 and y is B1 then ƒ1=Ƥ1x+q1y+r1 9) Rule2: if x is A2 and y is B2 then ƒ2=Ƥ2x+q2y+r2 And if for the non-fuzzy makers, we use the non-fuzzy maker of the average of the centers, the output will be as follows: 10) ) f=(w_1 f_1+w_2 f_2)/(w_1+w_2 ) w ̅_1 f_1+w ̅_2 f_2 stw ̅_1=w_1/(w_(1+) w_2 ),w ̅_2=w_2/(w_1+w_2 ) Layer Output: Layer 1: In this layer, inputs pass through membership functions: 11) O1,i=μAi(x)ƒor i=1,2 12) O1,i=μBi(x)ƒor i=3,4 The membership functions can fit any parametric function, which in most cases is chosen as Gaussian functions. Such as a generic bell function that is a set of parameters. Parameters of this layer are known as early parameters. 13) μA(x)=1/(1+|(x-c_i)/a_i |^(2b_i ) ) Layer 2: The output of this layer is the multiplication of the input signals, which is actually equivalent to the "if" part of the rules. 14) O2,i=wi=μAi (x) μBi (y),i=1,2 Layer 3: The output of this layer is normalization of the previous layer 15) O_(3,i)=w ̅_i=w_i/(w_1+w_2 ),i=1,2 Layer 4: Non-fuzzy average center maker 16) O4,i=w̅ i fi=w̅ i (pi x+qi y+ri Layer 5: 17) O_(5,i)=∑_i▒w ̅_i f_i=(∑_i▒〖w_i f_i 〗)/(∑_i▒w_i ) Now a network has been generated which is equivalent to the TSK fuzzy inference system (Fig. 2) [9]. The final output of the fuzzy system is shown in Fig. 3 [9]. Therefore, in this network, the Gaussian multiplication and Gaussian fuzzy, and the center average non-fuzzy maker were used. To train the adaptive neuro-fuzzy network parameters, a combination learning algorithm was used [9]. The REC (Receiver Operating Characteristic) curve was used to evaluate performance (sensitivity and test characteristics) in a system. The sensitivity of a test is its ability to detect a disease within a patient's population and, in fact, is the correct diagnosis of patients by the test. 21) TPF=TP/(TP+FN) Number of patients= (TP + FN) The extent of the characteristics of a test is its ability to detect the absence of a disease in a disease-free population; in fact, it can be said that the rate of the number of people who are not patient and tested by the test have been correctly recognized. 22) TNF=TN/(TN+FP) Number of non-patient persons = (TN + FP) The accuracy of the test is the correct rate of the results of a test. In fact, the number of people who are correctly diagnosed () is divided into the population of all individuals. 23) (TP+TN)/(TP+FP+TN+FN) The area under the ROC curve shows the system's efficiency. The largest area is one (Fig. 4). When the efficiency of a test increases, the ROC curve moves to the upper left corner of the graph, the area under the curve also increases and approaches 1 [10].
Initially, the color image turned into an image with gray levels. The red cells that were attached to the edge of the image were removed using the image processing method (Fig. 5). The location coordinates of each object were used to extract the following image with gray levels from the original image. Therefore, each gray underneath image contained only one cell. Then, each beneath of the image with gray levels was converted to binary image beneath using the otsu algorithm with the optimal threshold (Fig. 6).The size of the inner and outer diameters of the red cells was calculated. To remove unwanted volumes, volumes that were in the middle of 50% of mode were selected as acceptable volumes. The cross-sectional evaluation method was used to select and reverse the training data and test data. Therefore, the neuro-fuzzy network was tested once for all normal and abnormal data in the system. The training data included 21513 red cells (13835 normal data and 7678 abnormal data). The outer radius and radius of the red cell cavity were used as two input variables in the neuro-fuzzy network. For each entry, the Gaussian membership function is assigned, so the rule base consists of 36 bases. After 100 rounds of training, the mean squares of training error and test were 0.005 and 0.307 respectively. The form of the membership functions of the two inputs of the network after the ANFIS training and the procedure of the inputs and outputs of the network respectively are shown in Figures 7 to 10. Desired output and actual output of the ANFIS network based on the test data belonging to one category (each category including 4 normal people and 2 non-normal people) is shown in Fig. 11. The accuracy of the system and the area under the ROC curve were 96.6% and 99.5%, respectively. The numerical results from the implementation of the neuro-fuzzy network are shown in Table 1.
… [11]. In 2009, Apostolopoulos et al. presented a method for estimating the size of human red blood cells using images containing scattered light. The image data recovery process involved normalizing the image, applying a two-dimensional discrete cosine transform to the image, and applying a wavelet transform to the image. An RBF-NN (RBF-Neural Network) neural network, which estimated the geometric properties of red blood cells, was investigated. The proposed method has been used when three important RBC geometric features have been investigated using a database of 1575 simulated image with a threshold method [12].
The proposed method provides diagnostic capability using a drop of blood and is well suited to pathological images. The designed automatic system can be a convenient and affordable alternative to commonly used laboratory procedures. In addition, the proposed method can be a base for calculation of other parameters of blood test or CBC such as CBC, HCT, MCH, MCHC and the number of red blood cells, etc.
This article is the result of a joint research carried out between the Cellular-Molecular Research Center, the Stem Cells of the Sarem Medical Faculty, Center of Research, Sciences and Technology in Medicine of Tehran University, Amir Kabir University of Technology, and the Azad University of Science and Research Branch. All the relevant colleagues who helped us in conducting this study are appreciated.
TABLES and CHARTS
Show attach fileCITIATION LINKS
[1]Fauci AS, Braunwald E, Kasper DL, Hauser SL, Longo DL, Jameson JL et al. Harrison’s principles of internal medicine. 17th edition. New York: McGraw-Hill Medical Publishing Division; 2008. 2958p.
[2]Dahim P. Automated cell counter device (principles, calibration, quality control and error). Tehran: Seda Publish Center; 2009. pp.14-45. [Persian]
[3]Babazadeh Khameneh N, Arabalibeik H, Salehian P, Setayeshi S. Abnormal red blood cells detection using adaptive neuro-fuzzy system. Stud Health Technol Inform. 2012;173:30-4.
[4]Gonzalez RC, Woods RE. Digital image processing. 3rd edition. New Jersey: Pearson Education, Inc; 2008.
[5]Otsu N. A tlreshold selection method from gray-level histograms. IEEE Trans Sys Man Cyber. 1979;9(1):62-6.
[6]Soille P. Morphological image analysis: Principles and applications. Berlin: Springer; 2010.
[7]Haralick RM, Shapiro LG. Computer and robot vision. Boston: Addison-Wesley Longman Publishing; 1992. 630p.
[8]Torus. Wikipedia [Internet]. San Diego: 2001; [updated 2008 Jan 28; cited 2009 Dec]. Available from: https://en.wikipedia.org/wiki/Torus.
[9]Roger Jang JS. ANFIS: Adaptive-network-based fuzzy inference system. IEEE Trans Sys Man Cyber. 1993;23(3):665- 83.
[10]Fawcett T. An introduction to ROC analysis. Pattern Recogn Lett. 2006;27(8):861-74.
[11]Ghosh N, Buddhiwant P, Uppal A, Majumder SK, Patel HS, Gupta PK, editors. Simultaneous determination of size and refractive index of red blood cells by light scattering measurements. Appl Phys Lett. 2006;8(8).
[12]Apostolopoulos G, Tsinopoulos S, Dermatas E. Estimation of human red blood cells size using light scattering images. J Comput Method Sci Eng. 2009;1,2:19-30.
[2]Dahim P. Automated cell counter device (principles, calibration, quality control and error). Tehran: Seda Publish Center; 2009. pp.14-45. [Persian]
[3]Babazadeh Khameneh N, Arabalibeik H, Salehian P, Setayeshi S. Abnormal red blood cells detection using adaptive neuro-fuzzy system. Stud Health Technol Inform. 2012;173:30-4.
[4]Gonzalez RC, Woods RE. Digital image processing. 3rd edition. New Jersey: Pearson Education, Inc; 2008.
[5]Otsu N. A tlreshold selection method from gray-level histograms. IEEE Trans Sys Man Cyber. 1979;9(1):62-6.
[6]Soille P. Morphological image analysis: Principles and applications. Berlin: Springer; 2010.
[7]Haralick RM, Shapiro LG. Computer and robot vision. Boston: Addison-Wesley Longman Publishing; 1992. 630p.
[8]Torus. Wikipedia [Internet]. San Diego: 2001; [updated 2008 Jan 28; cited 2009 Dec]. Available from: https://en.wikipedia.org/wiki/Torus.
[9]Roger Jang JS. ANFIS: Adaptive-network-based fuzzy inference system. IEEE Trans Sys Man Cyber. 1993;23(3):665- 83.
[10]Fawcett T. An introduction to ROC analysis. Pattern Recogn Lett. 2006;27(8):861-74.
[11]Ghosh N, Buddhiwant P, Uppal A, Majumder SK, Patel HS, Gupta PK, editors. Simultaneous determination of size and refractive index of red blood cells by light scattering measurements. Appl Phys Lett. 2006;8(8).
[12]Apostolopoulos G, Tsinopoulos S, Dermatas E. Estimation of human red blood cells size using light scattering images. J Comput Method Sci Eng. 2009;1,2:19-30.