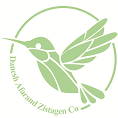
@2025 Afarand., IRAN
ISSN: 2251-8215 Sarem Journal of Reproductive Medicine 2017;1(3):97-104
ISSN: 2251-8215 Sarem Journal of Reproductive Medicine 2017;1(3):97-104
MCV Measurement of Abnormal Red Blood Cells Using Adaptive Neuro-Fuzzy System with Image Processing
ARTICLE INFO
Article Type
Original ResearchAuthors
Babazadeh Khameneh N. (1)Salehian P. (*)
Arabalibeik H. (2)
Setayeshi S. (3)
(*) Sarem Fertility & Infertility Research Center (SAFIR), Sarem Women’s Hospital, Tehran, Iran
(1) Islamic Azad University, Pardis Branch, Tehran, Iran
(2) Science and Technology in Medicine Research Center (RCSTIM), Tehran University of Medical Sciences, Tehran, Iran
(3) Energy Engineering & Physics Department, Amirkabir University of Technology, Tehran, Iran
Correspondence
Article History
Received: February 29, 2016Accepted: June 25, 2016
ePublished: August 15, 2017
ABSTRACT
Aims
Size, shape, and volume of Red Blood Cells are important factors in diagnosing blood-associated disorders such as iron deficiency and anemia. Every day, thousands of blood samples are tested by microscopes and automated cell counter devices in pathology laboratories around the world, which may be expensive and time-consuming. The objective of this study was to measure mean corpuscular volume of abnormal red blood cells using the adaptive neuro-fuzzy system with image processing.
Materials & Methods This study was conducted on 60 blood samples from the archive of pathology laboratory of Sarem hospital including 40 normal samples and 20 abnormal samples. Adaptive local thresholding and bounding box methods were used to extract the inner and outer diameters of red cells to calculate MCV. An adaptive-network-based fuzzy inference system was used to classify blood samples to normal and abnormal groups. In this method, normal and abnormal blood samples were examined using image processing techniques and MATLAB software.
Findings The Accuracy of the proposed method and area under the curve were found as 96.6% and 0.995%, respectively.
Conclusion The proposed method provides diagnostic capability using a drop of the blood sample and showed suitable performance on pathological images. The designed automatic system can be a convenient and cost effective alternative for common laboratory procedures. In addition, the method provides a basis for calculating other parameters of blood test or CBC such as mean cell hemoglobin, mean cell hemoglobin concentration, RDW, hematocrit, and red blood cell count.
Materials & Methods This study was conducted on 60 blood samples from the archive of pathology laboratory of Sarem hospital including 40 normal samples and 20 abnormal samples. Adaptive local thresholding and bounding box methods were used to extract the inner and outer diameters of red cells to calculate MCV. An adaptive-network-based fuzzy inference system was used to classify blood samples to normal and abnormal groups. In this method, normal and abnormal blood samples were examined using image processing techniques and MATLAB software.
Findings The Accuracy of the proposed method and area under the curve were found as 96.6% and 0.995%, respectively.
Conclusion The proposed method provides diagnostic capability using a drop of the blood sample and showed suitable performance on pathological images. The designed automatic system can be a convenient and cost effective alternative for common laboratory procedures. In addition, the method provides a basis for calculating other parameters of blood test or CBC such as mean cell hemoglobin, mean cell hemoglobin concentration, RDW, hematocrit, and red blood cell count.
Keywords:
Mean Cell Volume (MCV) ,
Adaptive Neural Network Base Fuzzy Inference System (ANFIS) ,
Image Processing ,
CITATION LINKS
[1]Fauci AS, Braunwald E, Kasper DL, Hauser SL, Longo DL, Jameson JL et al. Harrison’s principles of internal medicine. 17th edition. New York: McGraw-Hill Medical Publishing Division; 2008. 2958p.
[2]Dahim P. Automated cell counter device (principles, calibration, quality control and error). Tehran: Seda Publish Center; 2009. pp.14-45. [Persian]
[3]Babazadeh Khameneh N, Arabalibeik H, Salehian P, Setayeshi S. Abnormal red blood cells detection using adaptive neuro-fuzzy system. Stud Health Technol Inform. 2012;173:30-4.
[4]Gonzalez RC, Woods RE. Digital image processing. 3rd edition. New Jersey: Pearson Education, Inc; 2008.
[5]Otsu N. A tlreshold selection method from gray-level histograms. IEEE Trans Sys Man Cyber. 1979;9(1):62-6.
[6]Soille P. Morphological image analysis: Principles and applications. Berlin: Springer; 2010.
[7]Haralick RM, Shapiro LG. Computer and robot vision. Boston: Addison-Wesley Longman Publishing; 1992. 630p.
[8]Torus. Wikipedia [Internet]. San Diego: 2001; [updated 2008 Jan 28; cited 2009 Dec]. Available from: https://en.wikipedia.org/wiki/Torus.
[9]Roger Jang JS. ANFIS: Adaptive-network-based fuzzy inference system. IEEE Trans Sys Man Cyber. 1993;23(3):665- 83.
[10]Fawcett T. An introduction to ROC analysis. Pattern Recogn Lett. 2006;27(8):861-74.
[11]Ghosh N, Buddhiwant P, Uppal A, Majumder SK, Patel HS, Gupta PK, editors. Simultaneous determination of size and refractive index of red blood cells by light scattering measurements. Appl Phys Lett. 2006;8(8).
[12]Apostolopoulos G, Tsinopoulos S, Dermatas E. Estimation of human red blood cells size using light scattering images. J Comput Method Sci Eng. 2009;1,2:19-30.
[2]Dahim P. Automated cell counter device (principles, calibration, quality control and error). Tehran: Seda Publish Center; 2009. pp.14-45. [Persian]
[3]Babazadeh Khameneh N, Arabalibeik H, Salehian P, Setayeshi S. Abnormal red blood cells detection using adaptive neuro-fuzzy system. Stud Health Technol Inform. 2012;173:30-4.
[4]Gonzalez RC, Woods RE. Digital image processing. 3rd edition. New Jersey: Pearson Education, Inc; 2008.
[5]Otsu N. A tlreshold selection method from gray-level histograms. IEEE Trans Sys Man Cyber. 1979;9(1):62-6.
[6]Soille P. Morphological image analysis: Principles and applications. Berlin: Springer; 2010.
[7]Haralick RM, Shapiro LG. Computer and robot vision. Boston: Addison-Wesley Longman Publishing; 1992. 630p.
[8]Torus. Wikipedia [Internet]. San Diego: 2001; [updated 2008 Jan 28; cited 2009 Dec]. Available from: https://en.wikipedia.org/wiki/Torus.
[9]Roger Jang JS. ANFIS: Adaptive-network-based fuzzy inference system. IEEE Trans Sys Man Cyber. 1993;23(3):665- 83.
[10]Fawcett T. An introduction to ROC analysis. Pattern Recogn Lett. 2006;27(8):861-74.
[11]Ghosh N, Buddhiwant P, Uppal A, Majumder SK, Patel HS, Gupta PK, editors. Simultaneous determination of size and refractive index of red blood cells by light scattering measurements. Appl Phys Lett. 2006;8(8).
[12]Apostolopoulos G, Tsinopoulos S, Dermatas E. Estimation of human red blood cells size using light scattering images. J Comput Method Sci Eng. 2009;1,2:19-30.