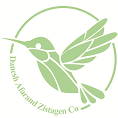
@2024 Afarand., IRAN
ISSN: 2251-8215 Sarem Journal of Reproductive Medicine 2017;1(3):105-112
ISSN: 2251-8215 Sarem Journal of Reproductive Medicine 2017;1(3):105-112
Analysis of the Factors on Intrauterine Insemination (IUI) Results by Clustering
ARTICLE INFO
Article Type
Original ResearchAuthors
Alizadeh S. (1)Asghari M. (*)
Hosseini M.K. (1)
(*) Information Technology Department, Computer Engineering Faculty, K. N. Toosi University of Technology, Tehran, Iran
(1) Information Technology Department, Computer Engineering Faculty, K. N. Toosi University of Technology, Tehran, Iran
Correspondence
Article History
Received: February 26, 2016Accepted: June 21, 2016
ePublished: August 15, 2017
ABSTRACT
Aims
Intra Uterine Insemination (IUI) is a medically-assisted reproduction technique (ART) enables infertile couples to achieve the successful pregnancy. Given the unpredictability of such techniques, many investigations have been done on the factors affecting the techniques. Data mining is one of the main tools that can help researchers to evaluate the factors. Data mining utilize the statistical methods along with the artificial intelligence (AI) to help different sciences including infertility science and research for interpreting the results and analyzes of data appropriately and extracting the hidden patterns and knowledge in the data. The objective of this study was to analyze the factors affecting IUI results by clustering.
Materials & Methods The IUI data were clustered utilizing the K-means )a clustering method in data mining). Davise-Buldian index was used to calculate the best number of clusters. The similar individuals were included in the same cluster and the success rates in those clusters were also measured.
Findings Some of the characteristics of individuals such as age, body mass index (BMI), type of infertility, the cause of infertility and etc. were effective factors on IUI success rate.
Conclusion Factors such as age, BMI, type of infertility, the cause of infertility and etc. can determine the success rate of the IUI method.
Materials & Methods The IUI data were clustered utilizing the K-means )a clustering method in data mining). Davise-Buldian index was used to calculate the best number of clusters. The similar individuals were included in the same cluster and the success rates in those clusters were also measured.
Findings Some of the characteristics of individuals such as age, body mass index (BMI), type of infertility, the cause of infertility and etc. were effective factors on IUI success rate.
Conclusion Factors such as age, BMI, type of infertility, the cause of infertility and etc. can determine the success rate of the IUI method.
CITATION LINKS
[1]Chakrabarti S, Ester M, Fayyad U, Gehrke J, Han J, Morishita S, et al. Data Mining Curriculum: A Proposal (Version 1.0) [Internet]. London: The community for data mining, data science and analytics, SIGKDD; 1999. [updated 2006 Apr 30; cited 2007 Dec 14]. Avilable from: www.kdd.org/exploration_files/CURMay06.pdf.
[2]Berry GT, Baker L, Kaplan FS, Witzleben CL. Diabetes-like renal glomerular disease in Fanconi-Bickel syndrome. Pediatr Nephrol. 1995;9(3):287-91.
[3]Han J, Kamber M. Data Mining: Concepts and Techniques. 2th ed. Burlington: Morgan Kaufmann; 2011.
[4]Saremi AT. Infertility Guideline. Tehran: Sarem Research Center; 2009. [Persian]
[5]Vahid Roudsari F, Ayati S, Mirzaeeyan S, Shakeri MT, Akhtardel H. Fertility outcome after IVF and related factors. J Gorgan Univ Med Sci. 2009;11(3):42-6. [Persian]
[6]Morales DA, Bengoetxea E, Larranaga P, Garcia M, Franco Y, Fresnada M, et al. Bayesian classification for the selection of in vitro human embryos using morphological and clinical data. Comput Methods Programs Biomed. 2008;90(2):104-16.
[7]Sohrabvand F, Shariat M, Fotoohi Ghiam N, Hashemi M. The relationship between number of transferred embryos and pregnancy rate in ART cycles. Tehran Univ Med J. 2009;67(2):132-6. [Persian]
[8]Gazanfari M, Alizadeh S, Teimourpur B. Data Mining and Knowledge Discovery. Tehran: Iran University of Science and Industry Press; 2011.
[9]Han L, Zhong Y, Huang B, Han L, Pan L, Xu X, et al. Sodium butyrate activates erythroid-specific 5-aminolevulinate synthase gene through Sp1 elements at its promoter. Blood Cells Mol Dis. 2008;41(2):148-53.
[10]Gonzalez T, Marggie D. A comparison in cluster validation techniques [Dissertation]. Puerto Rico: University of Puerto Rico; 2006.
[11]Witten IH, Frank E, Hall M. Data Mining: Practical Machine Learning Tools and Techniques. Burlington: Morgan Kaufmann; 2011.
[12]Guh RS, Wu TCJ, Weng SP. Integrating genetic algorithm and decision tree learning for assistance in predicting in vitro fertilization outcomes. Expert Syst Appl. 2011;38(4):4437-49.
[13]Jurisica I, Mylopoulos J, Glasgow J, Shapiro H, Casper RF. Case-based reasoning in IVF: Prediction and knowledge mining. Artif Intell Med. 1998;12(1):1-24.
[14]Cai Qf, Wan F, Huang R, Zhang HW. Factors predicting the cumulative outcome of IVF/ICSI treatment: A multivariable analysis of 2450 patients. Hum Reprod. 2011;26(9):2532-40.
[2]Berry GT, Baker L, Kaplan FS, Witzleben CL. Diabetes-like renal glomerular disease in Fanconi-Bickel syndrome. Pediatr Nephrol. 1995;9(3):287-91.
[3]Han J, Kamber M. Data Mining: Concepts and Techniques. 2th ed. Burlington: Morgan Kaufmann; 2011.
[4]Saremi AT. Infertility Guideline. Tehran: Sarem Research Center; 2009. [Persian]
[5]Vahid Roudsari F, Ayati S, Mirzaeeyan S, Shakeri MT, Akhtardel H. Fertility outcome after IVF and related factors. J Gorgan Univ Med Sci. 2009;11(3):42-6. [Persian]
[6]Morales DA, Bengoetxea E, Larranaga P, Garcia M, Franco Y, Fresnada M, et al. Bayesian classification for the selection of in vitro human embryos using morphological and clinical data. Comput Methods Programs Biomed. 2008;90(2):104-16.
[7]Sohrabvand F, Shariat M, Fotoohi Ghiam N, Hashemi M. The relationship between number of transferred embryos and pregnancy rate in ART cycles. Tehran Univ Med J. 2009;67(2):132-6. [Persian]
[8]Gazanfari M, Alizadeh S, Teimourpur B. Data Mining and Knowledge Discovery. Tehran: Iran University of Science and Industry Press; 2011.
[9]Han L, Zhong Y, Huang B, Han L, Pan L, Xu X, et al. Sodium butyrate activates erythroid-specific 5-aminolevulinate synthase gene through Sp1 elements at its promoter. Blood Cells Mol Dis. 2008;41(2):148-53.
[10]Gonzalez T, Marggie D. A comparison in cluster validation techniques [Dissertation]. Puerto Rico: University of Puerto Rico; 2006.
[11]Witten IH, Frank E, Hall M. Data Mining: Practical Machine Learning Tools and Techniques. Burlington: Morgan Kaufmann; 2011.
[12]Guh RS, Wu TCJ, Weng SP. Integrating genetic algorithm and decision tree learning for assistance in predicting in vitro fertilization outcomes. Expert Syst Appl. 2011;38(4):4437-49.
[13]Jurisica I, Mylopoulos J, Glasgow J, Shapiro H, Casper RF. Case-based reasoning in IVF: Prediction and knowledge mining. Artif Intell Med. 1998;12(1):1-24.
[14]Cai Qf, Wan F, Huang R, Zhang HW. Factors predicting the cumulative outcome of IVF/ICSI treatment: A multivariable analysis of 2450 patients. Hum Reprod. 2011;26(9):2532-40.